Google Research has introduced LANISTR, a cutting-edge framework for integrating structured and unstructured data for multimodal learning. This innovative system can process diverse data types, including images, text, time series, and tabular data, to generate class predictions.
LANISTR addresses key challenges such as overfitting and data heterogeneity by using attention-based architectures and cross-attention mechanisms for effective data fusion. Notably, it performs well even with incomplete data inputs, making it highly robust for real-world applications in healthcare and retail.
Model Architecture and Training
LANISTR uses modality-specific encoders for different data types and a multimodal encoder-decoder module for fusion. This architecture uses attention-based methods to deal with the complexity of diverse data inputs. Training of the model includes unimodal masking targets and a novel similarity-based multimodal masking loss, ensuring effective learning despite missing modalities.
Performance and Applications
Tested on healthcare and retail datasets, LANISTR has shown remarkable improvements in prediction, even with minimal labeled data. For example, it achieved high accuracy in predicting mortality on the MIMIC-IV dataset and product ratings on Amazon review data, outperforming several competitive baselines.
LANISTR represents a significant advancement in multimodal learning, demonstrating the potential to handle a wide range of data types and applications. Its robust architecture and innovative training methods position it as a leading framework for future AI developments.
For more details, see the full research paper here.
You May Also Like
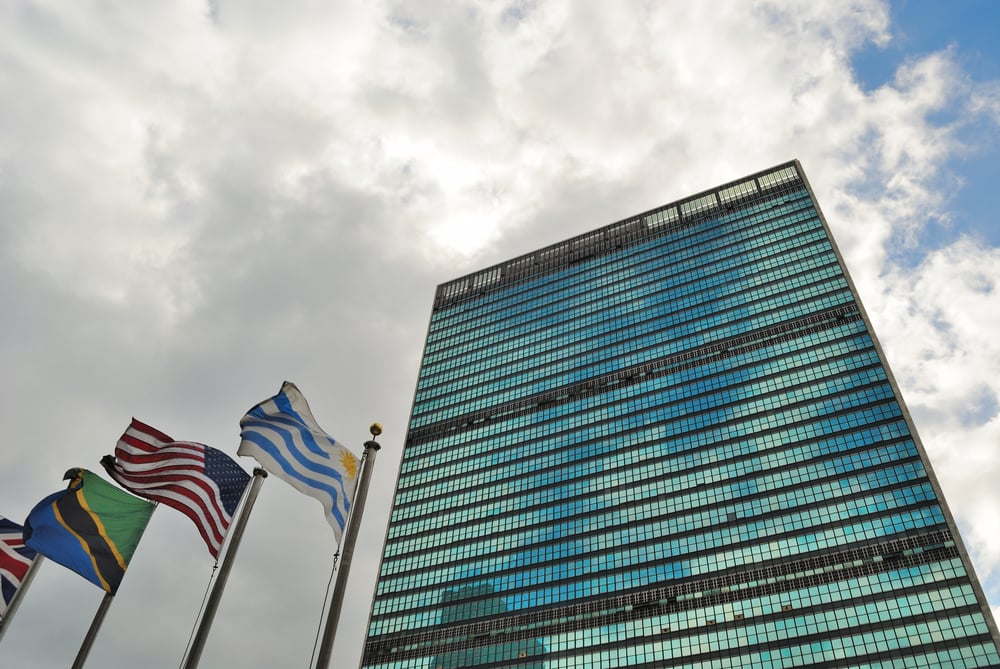
Council of Europe Adopts AI Treaty

OpenAI’s newest model is GPT-4o
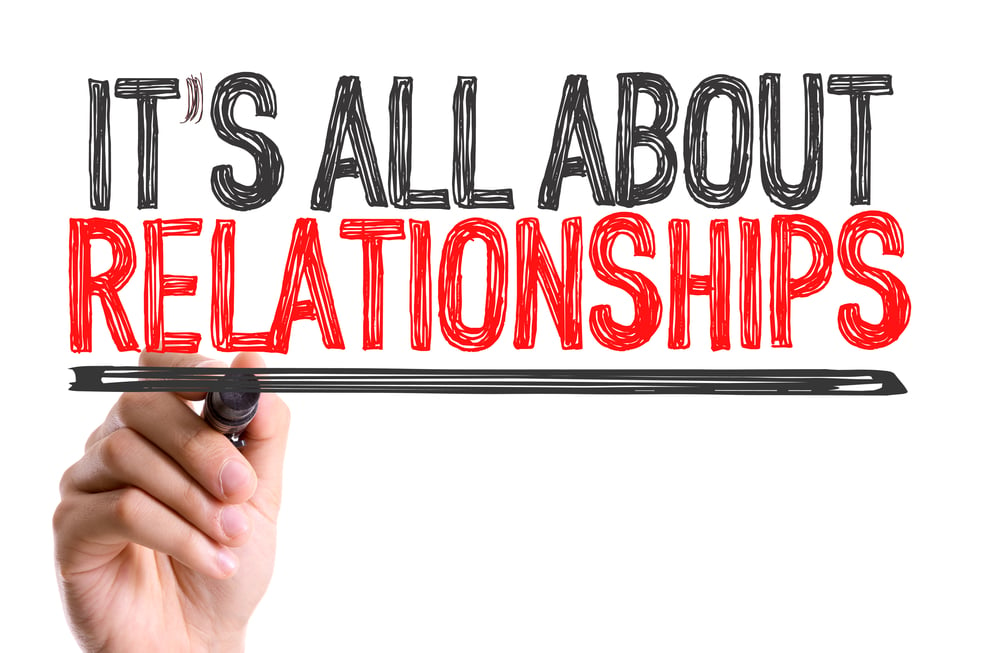